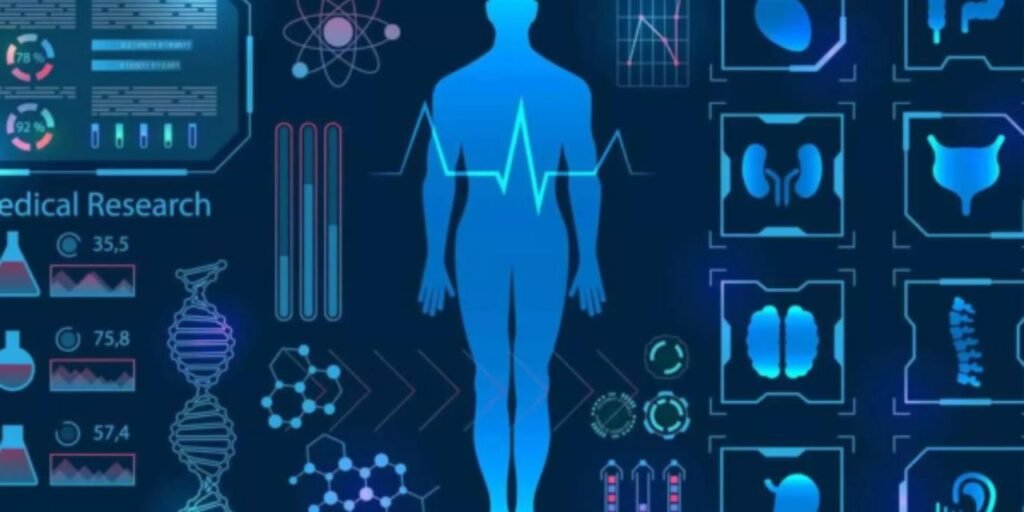
The global AI in Pharma and Biotech market, valued at US$ 859.0 million in 2022, is poised for significant growth over the coming years. Projected to advance at a robust CAGR of 11.2% from 2023 to 2031, the market is expected to surpass US$ 2.0 billion by 2031. This expansion is driven by the increasing adoption of AI technologies in healthcare, which enhance diagnostic accuracy, speed, and efficiency, ultimately improving patient outcomes and revolutionizing cancer detection and treatment.
AI in Pharma and Biotech: Revolutionizing Early Detection and Treatment
The integration of artificial intelligence (AI) into cancer diagnostics is a groundbreaking advancement that promises to revolutionize the early detection, diagnosis, and treatment of various cancer types. By leveraging the power of machine learning algorithms and vast datasets, AI can analyze medical data with unprecedented accuracy and speed, significantly improving patient outcomes and transforming the landscape of oncology.
The Need for AI in Pharma and Biotech
Cancer remains one of the leading causes of death worldwide. Early detection is critical for improving survival rates, as treatment is generally more effective during the initial stages of the disease. Traditional diagnostic methods, while effective, are often time-consuming, costly, and subject to human error. Radiologists and pathologists, despite their expertise, can sometimes miss subtle signs of cancer due to fatigue or the sheer volume of data they must process.
AI addresses these challenges by offering tools that can process and analyze medical images and data more efficiently and accurately than humans. These tools are not meant to replace medical professionals but to augment their capabilities, providing a second pair of eyes that can catch what might otherwise be overlooked.
How AI Works in Cancer Diagnostics
AI in Pharma and Biotech primarily employs machine learning, a subset of AI where algorithms learn from large datasets to identify patterns and make predictions. There are several key areas where AI is making a significant impact:
Medical Imaging Analysis:
Radiology: AI algorithms can analyze images from X-rays, CT scans, MRI scans, and mammograms with remarkable precision. For instance, AI can detect lung nodules in CT scans, often identifying malignant growths at a stage when they are still small and more treatable.
Pathology: AI systems can examine tissue samples and recognize cancerous cells. Digital pathology, where tissue slides are digitized and analyzed by AI, enhances the accuracy and speed of diagnoses. AI can identify patterns that might be too subtle for the human eye, such as early-stage melanoma in skin biopsies.
Genomic Data Analysis:
AI can analyze genomic data to identify mutations associated with various cancers. By examining the genetic makeup of cancer cells, AI can help in predicting how a cancer might behave and which treatments are likely to be most effective. This personalized approach to cancer treatment is known as precision oncology.
Predictive Analytics:
AI algorithms can predict the likelihood of cancer recurrence and patient survival rates by analyzing historical data and identifying risk factors. This helps in tailoring follow-up care and treatment plans to individual patients, improving overall outcomes.
Natural Language Processing (NLP):
NLP, a branch of AI, is used to extract relevant information from unstructured data such as clinical notes, research articles, and patient records. By processing this information, AI can provide insights that aid in diagnosis and treatment planning.
Advantages of AI in Pharma and Biotech
The integration of AI into cancer diagnostics offers numerous benefits:
Increased Accuracy:
AI systems can analyze large volumes of data with high precision, reducing the risk of false positives and false negatives. Studies have shown that AI can match or even surpass the diagnostic accuracy of human experts in some cases.
Efficiency:
AI significantly reduces the time required to analyze medical images and data, allowing for quicker diagnosis and treatment. This is particularly important in high-volume settings where medical professionals are overwhelmed by the number of cases.